
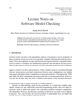
Objective. Injuries caused by falls of elderly people are a common worldwide problem and ageing of population will even further increase related burdens and costs. In this paper we present a monitoring system that detects dangerous actions of elderly and raises an alarm when necessary. Such a system would improve the quality of life for elderly and reduce costs in healthcare in general. The main requirements in its design are that the system can perform in real time with a high detection rate using realistic data, and to make it affordable so that it can be used as a healthcare monitoring system.
Main content. Our system is equipped with a single wide angle camera mounted on the ceiling of an elderly home. This gives a topview image of the environment resulting in a clear map of household objects without any occlusions. The main idea is to monitor the motion information of elderly and to model actions as change of motion or poses in time that lead to a specific action. After background subtraction, the motion information is extracted using the Motion History Images method and analyzed to detect important actions. We propose to model actions as the shape deformations of the motion history image in time. Every action is defined with the specific shape parameters taken at several moments in time. Shape models are extracted in offline analysis and used for comparison in room monitoring. For testing, we used a real elderly home environment in which we monitored in various environmental conditions a total of three different actions that are dangerous for elderly people: “Walking”, “Bending” and “Getting Up”.
Results. The obtained results from observing real elderly household show that our system can detect dangerous actions in a real time with high recognition rates. It can separate Walking, Bending and Standing Up actions with detection rates up to 94%.
Conclusion. Our system is able to detect multiple actions by analyzing the size and shape information of the motion history image. Because of the high recognition rates we can conclude that the presented method has a large potential to be further implemented in a real healthcare monitoring system which will increase the safety of elderly and improve their quality of life. We are now gathering data from various elderly homes to test our system, further improve detection rates and get a better understanding of actions that are happening. The presented system performed both training and testing on data obtained form a same household environment. We are now working on a design of a more advanced algorithm that will be able to transfer models learned in one environment to other households, which will allow a wider applicability of our system.