
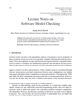
Reconstructing a 3D face from a single face image is a challenging problem in a wide range of applications. Due to the lack of a large number of 3D face datasets with ground truth, previous methods usually adopt weakly supervised learning methods. However, most methods only utilize pixel level information, which causes the convolutional neural network models to easily fall into local minima. This paper proposes a novel method of 3D face reconstruction and dense face alignment based on a single face image under unknown pose, expression and illumination. We not only consider the difference between the input face image and the rendered image at the pixel level, but also consider their difference in the deep feature space. First, a 3D face model is constructed from a single face image by using a parameterized face model. Then, the 3D face model is rendered to a 2D plane through a differentiable renderer. Next, the correspondences between the input face image and the rendered image in the pixel space and the deep feature space are established, respectively. Finally, our model is trained by back propagation. Experiments on AFLW2000-3D and AFLW-LFPA show that the proposed method outperforms existing approaches in both 3D face reconstruction and dense face alignment.