
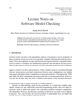
We tested the value of adding data from the operating room to models predicting in-hospital death. We assessed model performance using two metrics, the area under the receiver operating characteristic curve (AUROC) and the area under the precision-recall curve (AUPRC), to illustrate the differences in information they convey in the setting of class imbalance. Data was collected on 74,147 patients who underwent major noncardiac surgery and 112 unique features were extracted from electronic health records. Sets of features were incrementally added to models using logistic regression, naïve Bayes, random forest, and gradient boosted machine methods. AUROC increased as more features were added, but changes were small for some modeling approaches. In contrast, AUPRC, which reflects positive predicted value, exhibited improvements across all models. Using AUPRC highlighted the added value of intraoperative data, not seen consistently with AUROC, and that with class imbalance AUPRC may serve as the more clinically relevant criterion.