
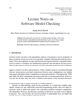
Traditional collaborative filtering algorithm has a shortcoming—it assigns all items with equal importance, which can result in excessive frequency in recommending hot items and reduced novelty and accuracy. Thus, we propose a collaborative filtering recommendation model based on fusion of item correlation-weighted and optimal-weighted. First we use correlation-weighted method to find the best threshold which could ensure the stability of algorithm under the sparse condition. Then, we fusion the correlation-weighted method with item optimal-weighed strategy on the condition of selecting the optimal threshold. Targeting final mean absolute error (MAE) of collaborative filtering, we introduce the weight value in the prediction process to enhance the performance of mining items in which users are really interested. Theoretically, fusion model can effectively overcome the problem in traditional collaborative filtering algorithm, and combine the advantage of correlation-weighted and item optimal-weighted. Experiment results in MovieLens-100K data set show that MAE of the a collaborative filtering recommendation model based on fusion of item correlation-weighted and optimal-weighted is lower than traditional collaborative algorithm, item optimal-weight collaborative algorithm, correlation-weight collaborative algorithm and variance-weight collaborative algorithm. The model we proposed reduces the item average popularity, increases the coverage and improves the recall rate and more novelty is achieved.