
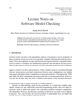
This paper contributes to the discourse on how information technologies can empower individuals to effectively manage their privacy while contributing to the emergence of balanced personal data ecosystems with increased trust between all stakeholders. We motivate our work through the prism of privacy issues in online social networks (OSN) acknowledging the fact that OSNs have become important platforms for information sharing. We note that on OSN platforms, individuals share very intimate details about different aspects of their lives, often lacking awareness about and understanding of the degree of accessibility/visibility of information they share as well as what it may implicitly reveal about them. Furthermore, service providers and other entities participating on OSN platforms are increasingly relying on profiling and analytics to find and extract valuable, often hidden patterns in large collections of data about OSN users. These issues have caused serious privacy concerns. In the light of such issues and concerns, we argue that protecting privacy online implies ensuring information and risk awareness, active control and oversight by the users over the collection and processing of their data, and support in assessing the trustworthiness of peers and service providers. Towards these goals, we propose the Personal Information Dashboard (PID), a system that relies on usable automation tools and intuitive visualizations to empower end-users to effectively manage both their personal data and their self-presentations. With the PID, the user is able to monitor and visualize her social networking footprint across multiple OSN domains. To enable this, the PID aggregates personal data from multiple sources and links the user's multiple OSN profiles. Leveraging various inference and correlation models as well as machine learning techniques, the PID empowers users to assess and understand the level of privacy risk they are facing when sharing information on OSNs. Based on outputs of the underlying prediction and learning methods, the PID can suggest to the user corrective options aimed at reducing risks of unintended information disclosure. We present a prototype implementation demonstrating the feasibility of our proposal.