
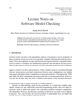
Conventionally, Kansei engineering relies heavily on the intuition of the person who uses the method in clustering the Kansei. As a result, the selection of Kansei adjectives may not be consistent with the consumer's opinions. Nevertheless, to obtain a consumer-consistent result, all of the collected Kansei adjectives (usually hundreds) might need to be evaluated by every survey participant, which is impractical in most design cases. Accordingly, a Kansei clustering method based on design structure matrix (DSM) and graph decomposition (GD) is proposed in this work. The method breaks the Kansei adjectives down into a number of subsets for the ease of management among the survey participants. In so doing, each participant deals with only a portion of the collected words and the subsets are integrated using a DSM-based algorithm for an overall Kansei clustering result. In order to differentiate the groups in the combined DSM further, graph decomposition (GD) is used to yield non-exclusive Kansei clusters. The hybrid approach, i.e., using DSM and GD, is able to handle the Kansei clustering problem. A case study on cordless battery drills is used to illustrate the proposed approach. The obtained results are compared and discussed.