
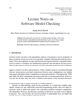
A new data mining method of Support Vector Machines (SVM) is applied on the classification of rock mass in tunnels. SVM is a novel powerful leaning method that based on Statistical Learning Theory. SVM can solve small-sample learning problems better than neural network. Eight qualitative indexes, such as rock layer thickness, rock mass structure, inlay condition, weathering condition, groundwater characteristic, joint condition, hammer knocking sound and ground stress, are chose as the judge factors. Twenty two data samples from Niba mountain tunnel are used to train the SVM with different kernels, such as linear, quadratic and polynomial kernel. Use the mapping relationship between judge factors and rock mass classes which the SVM provided class-unknown data samples of rock mass can be discriminated. Ten samples of test data are used to test the accuracy of SVM with different kernels. The result of the classification shows that SVM with polynomial kernel have a high accuracy when classify the rock mass. A website that based on ASP.NET and database has been developed to store the geological data from the tunnel, and using these data the background program which based on SVM can calculate the class that the surrounding rocks of the tunnel belong to, and the result can be show on the web page for engineers to use. So this is an intelligent classification of rock mass method that can be applied to classify rock mass in tunnels and is also a kind of information technologies in Geo-Engineering.