
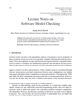
The Hyperion sensor is unique because it is the only high spectral resolution hyperspectral sensor on a satellite platform. The hyperspectral capability of the sensor could potentially allow for classifications of increased accuracy, in comparison to those produced from multispectral data. This study evaluates the capability of Hyperion data for discriminating land-cover classes in the Anopoli region (southwest Crete, Greece), through different classification techniques and spectral unmixing procedure. Preprocessing of Hyperion data is essential before any analysis takes place, and it includes interpolation of the wavelengths to a common set, noise reduction by using the minimum noise fraction (MNF), and other commonly applied corrections. Land-cover classifications were performed on the Hyperion satellite data through the Spectral Angle Mapper (SAM) pixel-based technique, as well as the object-based technique. In order to take advantage of the hyperspectral data of Hyperion, and overcome the problem of low spatial resolution, non-linear spectral unmixing approaches were employed, by using artificial neural network (ANN). The spectral end-members used in those approaches were constructed from QuickBird data. All the results were evaluated using the ground truth data derived from high spatial resolution imagery (QuickBird) and field data. All classification results mainly appeared to suffer from the relatively low spatial resolution of the Hyperion sensor and the spectral similarity among many land cover classes. The results show that the object-based classification outperformed the pixel-based classification and achieved the highest overall accuracy. The pixel-based classification and ANN results were highly dependent on the extent that the end-members were representative of the class. However, the pixel-based technique was very successful in differentiating land cover classes with dense vegetation cover.