
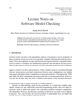
Human Pose Estimation (HPE) is a critical Computer Vision task with applications ranging from video surveillance to medical rehabilitation. Despite recent advancements in Deep Learning, HPE still faces challenges such as occluded keypoints, variable lighting conditions, and high computational demands. To address these issues, we present the Attention-Based Multi-Scale, Context-Aware Feature Integration into PoseResNet for Coordinate Classification (AMSF-PRNetCC). Our framework enhances the traditional ResNet architecture by incorporating CoordConv2d layers, depthwise separable convolutions, and novel attention mechanisms including Spatial-Enhanced Channel Attention (SECA) and Squeeze-and-Excitation (SE). We introduce a Context-Aware Feature Pyramid Network (CAFPN) with Dual Mask Global Context Blocks (DMGCB) to efficiently handle multi-scale information. The model culminates in Multi-Layer Perceptron (MLP) stages for precise keypoint coordinate classification. Evaluations on the COCO dataset demonstrate that AMSF-PRNetCC significantly outperforms existing 2D HPE methods in both accuracy and computational efficiency. Our approach achieves state-of-the-art results while requiring fewer computational resources, marking a substantial advancement in the field of HPE.