
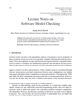
In this paper, we propose Long-Short Term Memory (LSTM)-based mosquito’s genus classification, in which the time-frequency features are extracted from the wingbeat sound of mosquitos of three genera, Aedes, Anopheles and Culex. The extracted features are fed into the proposed LSTM-based classifier. We evaluated three time-frequency features, which are: Mel Spectrogram, Log-Mel spectrogram, and Mel-frequency Cepstral Coefficients (MFCC). The proposed scheme is composed by two LSTM layers and one Fully Connected layer connected to a SoftMax activation function. The classification accuracies using the three features are 92.97(±0.2)%, 96.71(±0.2)% and 96.65(±0.2)%, respectively. The Area Under Curve (AUC) of the Receiver Operating Characteristics (ROC) for each feature are also obtained, which are 0.9944, 0.9986 and 0.9987, respectively. The proposed classifier requires approximately 62,000 trainable parameters. This number is much smaller than that required for the state-of-arts CNNs, such as AlexNet and Vgg16. This compact configuration of the proposed scheme takes advantage of the mobile and IoT implementation, because the number of trainable parameters is directly proportional to the amount of memory and CPU required.