
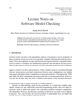
Preparation of land cover / land use maps for large areas, based on automatic classification of high-resolution satellite data is the objective of many application programmes, e.g. GSE Land Monitoring Services. The crucial point for this kind of activity is to apply optimal classification approach, which will ensure high class recognition accuracy and classification repeatability. Among different approaches object-oriented approach seems to give the best results, as it allows to use various spectral and non-spectral features in the classification process and enables to have more control of the final map accuracy.
The presented paper demonstrates the method, developed within object-oriented approach, called rule-based classification. In this method SPOT satellite image with 20-meter resolution was analyzed in eCognition environment, assuming that Standard Nearest Neighbour classifier, based on selected sample objects, will not be applied. Instead of it, contents of SPOT satellite image was characterized by various spectral / texture parameters describing directly particular land cover / land use classes, as well as by pre-defined functions, determined on the basis of graphical analysis of feature space constructed for particular terrain objects.
The classification process begins with recognition of water class, whose objects were delineated using multi-resolution segmentation. For the remaining land cover classes new segmentation is prepared. In turn, general forest class and urban class are discriminated; in the next stage both classes are divided into more specified categories. Consequently, broad agricultural and grassland classes are determined, being next divided into more detailed information classes. In total 13 land cover / land use categories were discriminated in the presented work. Final classification map has been prepared using aggregation procedure, in order to obtain map resolution fulfilling 4ha size of Minimum Mapping Unit. Overall accuracy of all classes checked through verification process reached 89.1% with Kappa statistic 0.87.