
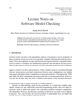
Knowledge Graphs (KGs) are relational knowledge bases that represent facts as a set of labelled nodes and the labelled relations between them. Their machine learning counterpart, Knowledge Graph Embeddings (KGEs), learn to predict new facts based on the data contained in a KG – the so-called link prediction task. To date, almost all forms of link prediction for KGs rely on some form of embedding model, and KGEs hold state-of-the-art status for link prediction. In this paper, we present TWIG-I (Topologically-Weighted Intelligence Generation for Inference), a novel link prediction system that can represent the features of a KG in latent space without using node or edge embeddings. TWIG-I shows mixed performance relative to state-of-the-art KGE models – at times exceeding or falling short of baseline performance. However, unlike KGEs, TWIG-I can be natively used for transfer learning across distinct KGs. We show that using transfer learning with TWIG-I can lead to increases in performance in some cases both over KGE baselines and over TWIG-I models trained without finetuning. While these results are still mixed, TWIG-I clearly demonstrates that structural features are sufficient to solve the link prediction task in the absence of embeddings. Finally, TWIG-I opens up cross-KG transfer learning as a new direction in link prediction research and application.