
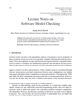
The usage of Named Entity Recognition tools on domain-specific corpora is often hampered by insufficient training data. We investigate an approach to produce fine-grained named entity annotations of a large corpus of Austrian court decisions from a small manually annotated training data set. We apply a general purpose Named Entity Recognition model to produce annotations of common coarse-grained types. Next, a small sample of these annotations are manually inspected by domain experts to produce an initial fine-grained training data set. To efficiently use the small manually annotated data set we formulate the task of named entity typing as a binary classification task – for each originally annotated occurrence of an entity, and for each fine-grained type we verify if the entity belongs to it. For this purpose we train a transformer-based classifier. We randomly sample 547 predictions and evaluate them manually. The incorrect predictions are used to improve the performance of the classifier – the corrected annotations are added to the training set. The experiments show that re-training with even a very small number (5 or 10) of originally incorrect predictions can significantly improve the classifier performance. We finally train the classifier on all available data and re-annotate the whole data set.