
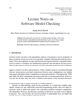
Background:
This study focuses on the development of a neural network model to predict perceived sleep quality using data from wearable devices. We collected various physiological metrics from 18 participants over four weeks, including heart rate, physical activity, and both device-measured and self-reported sleep quality.
Objectives:
The primary objective was to correlate wearable device data with subjective sleep quality perceptions.
Methods:
Our approach used data processing, feature engineering, and optimizing a Multi-Layer Perceptron classifier.
Results:
Despite comprehensive data analysis and model experimentation, the predictive accuracy for perceived sleep quality was moderate (59%), highlighting the complexities in accurately quantifying subjective sleep experiences through wearable data. Applying a tolerance of 1 grade (on a scale from 1-5), increased accuracy to 92%.
Discussion:
More in-depth analysis is required to fully comprehend how wearables and artificial intelligence might assist in understanding sleep behavior.