
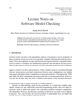
Making health data available for secondary use enables innovative data-driven medical research. Since modern machine learning (ML) methods and precision medicine require extensive amounts of data covering most of the standard and edge cases, it is essential to initially acquire large datasets. This can typically only be achieved by integrating different datasets from various sources and sharing data across sites. To obtain a unified dataset from heterogeneous sources, standard representations and Common Data Models (CDM) are needed. The process of mapping data into these standardized representations is usually very tedious and requires many manual configuration and refinement steps. A potential way to reduce these efforts is to use ML methods not only for data analysis, but also for the integration of health data on the syntactic, structural, and semantic level. However, research on ML-based medical data integration is still in its infancy. In this article, we describe the current state of the literature and present selected methods that appear to have a particularly high potential to improve medical data integration. Moreover, we discuss open issues and possible future research directions.