
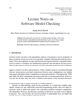
Multimorbidity, having a diagnosis of two or more chronic conditions, increases as people age. It is a predictor used in clinical decision-making, but underdiagnosis in underserved populations produces bias in the data that support algorithms used in the healthcare processes. Artificial intelligence (AI) systems could produce inaccurate predictions if patients have multiple unknown conditions. Rural patients are more likely to be underserved and also more likely to have multiple chronic conditions. In this study, data collected during the course of care in a centrally located academic hospital, multimorbidity decreased with rurality. This decrease suggests a bias against rural patients for algorithms that rely on diagnosis information to calculate risk. To test preprocessing to address bias in healthcare data, we measured the amount of discrimination in favor of metropolitan patients in the classification of multimorbidity. We built a model using the biased data to test optimum classification performance. A new unbiased training data set and model were created and tested against unaltered validation data. The new model’s classification performance on unaltered data did not diverge significantly from the performance of the initial optimal model trained on the biased data suggesting that bias can be removed with preprocessing.