
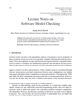
In response to the challenges of limited dataset size and low image resolution in steel surface defect datasets, leading to suboptimal recognition accuracy and overall performance of deep learning algorithms, this paper proposes a deep learning-based model for steel surface defect detection. Leveraging the AFPAN (Asymptotic Feature Pyramid Network) as the fusion network, the model utilizes the YOLOv8 backbone network for feature extraction. Additionally, it introduces the SPD-Conv (Space-to-Depth) block and the EffectiveSE (Effective Squeeze-and-Excitation) attention mechanism, specifically designed for low-resolution images. While maintaining a low parameter count, the model significantly enhances performance. Compared to algorithms such as SSD (Single Shot MultiBox Detector) and DETR (DEtection TRansformer), our algorithm achieves a more balanced Precision and Recall, without exhibiting a noticeable imbalance between the two metrics. Additionally, it surpasses algorithms like RT-DETR (Real Time-DEtection TRansformer) in terms of accuracy and comprehensiveness. In contrast to the YOLO (You only look once) series, the improved network exhibits optimal performance in smaller models, achieving a 4.8% precision improvement and a 3.2% increase in mAP (mean average precision). Experimental results on the NEU-DET (NEU surface defect database) dataset affirm that, compared to the YOLOv8s model, our proposed model excels in accurately and comprehensively identifying defect positions and edges in defect detection.