
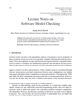
This paper introduces methods for efficiently classifying mangoes intended for export, a market valued at approximately 4,500 million baht annually. The classification process is divided into two primary components: size categorization and quality assessment. The first phase involves categorizing mango sizes into four distinct categories (M, 1L, 2L, 3L), while the second phase focuses on assessing mango quality, particularly detecting anthracnose—a prevalent disease affecting mangoes. This detection is achieved through image processing techniques, where images are converted into binary representations and analyzed using both Artificial Neural Networks (ANNs) and Convolutional Neural Networks (CNNs). Comparative analysis reveals that CNN outperforms ANN in size classification, achieving a remarkable train accuracy of 99.16% and a test accuracy of 88.0%. Furthermore, in quality classification, CNN demonstrates a train accuracy of 91.0% and a test accuracy of 88.0%. These findings underscore the effectiveness of CNN techniques in accurately classifying mangoes for export, thereby facilitating improved quality control and market competitiveness.