
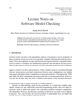
Knowledge graphs (KGs) are essential in human-centered AI by reducing the need for extensive labeled machine-learning datasets, enhancing retrieval-augmented generation, and facilitating explanations. However, modern KG construction has evolved into a complex, semi-automated process, increasingly reliant on opaque deep-learning models and a multitude of heterogeneous data sources to achieve the scale and meet the requirements of downstream applications. As such, the KG lifecycle poses the same risks as any other AI context; designing AI assistants for KG construction requires a deeper understanding of emerging knowledge engineering practices, with a greater focus on automation with human oversight, as well as bespoke solutions to ensure transparency, accountability, fairness, and other trustworthy AI concerns. This chapter undertakes a comprehensive exploration of trustworthy KG construction through a systematic literature review combined with a series of use cases and user studies. It highlights tasks in the KG lifecycle where automation is underexplored, introduces new AI assistants for such KG tasks, and discusses gaps in research, datasets, and capabilities to use AI responsibly.