
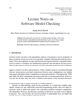
Object detection often struggles when applied to low-resource, domain-specific datasets. This challenge is exacerbated when dealing with sports-related data such as rugby, where fast-paced gameplay and tackles result in frequent instances of motion blur and occlusion, representing a substantial domain-shift from widely available pre-trained models. Given the high cost of manual labelling, we seek to determine whether we can minimise the number examples needed for fine-tuning by identifying implausible label classifications made by pre-trained object detection models. We do this using a coarse-grained labelling approach in the absence of detailed ground truth bounding boxes, allowing us to determine whether a label is implausible within the context of a rugby pitch. This is done to maximize the information provided by each example used for fine-tuning with the goal of minimizing the number of examples needed. Our results show that using pool-based, single-step uncertainty sampling to select examples from a subset of frames with implausible labels improves the model performance. More specifically, we show that fine-tuning on frames with the lowest confidence scores first can lead to greater performance after roughly 30 examples.