
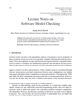
The pharmaceutical industry must adhere to rigorous regulations to meet specific quality standards. Additionally, the intricate nature of pharmaceutical manufacturing processes and long time to production necessitates timely detection of batch failures. AI/ML models are used for predictive maintenance in an automated and data-driven manner to detect these failures and aid timely intervention. However, these models require substantial amount of data for model training. This can lead to extended time-to-value before a predictive monitoring system can be deployed for any new process due to long process lead times. The current research proposes COSYNE, a generative AI-based approach to generate manufacturing digital twin, reducing the model development time by augmenting synthetic data with real data. The proposed solution is validated on a large pharmaceutical company’s batch manufacturing dataset, and the results are benchmarked across multiple dimensions of generation quality. Empirical results demonstrate that the proposed COSYNE outperforms the state-of-the-art approach by 2-3 times on average across all the generation quality metrics. Moreover, COSYNE enhances downstream AI/ML performance significantly through data augmentation and reduces time-to-value by creating high-fidelity digital twins with only 10% of real data and still achieve similar performance as current baseline trained on entire real data.