
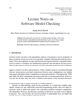
As modern neural network training and fine-tuning requires a lot of computational resources, there is a huge demand for novel, specialized algorithms for efficient and cost-effective training procedures. Aggressive Loss-based Elimination of Samples (ALOE) is an innovative method that operates with training samples based on losses obtained from a currently trained model or a pre-trained one. ALOE is designed to accelerate the fine-tuning process of Large Language Models and is perfectly integrated with the state-of-the-art Parameter-Efficient Fine-Tuning method LoRA. ALOE is a two-stage fine-tuning acceleration method. The two stages of ALOE are called offline and online. The proposed method is based on the idea that reducing the number of samples due to a certain rule decreases the number of training steps, thus reducing the overall fine-tuning time for LLM. This reduction allows to either get a fine-tuned version of the model faster or to perform more training iterations within the same time period as the fine-tuning baseline (ALOE Max). The ALOE (Offline) performs dataset reduction before the fine-tuning starts, while the ALOE (Online) selects the training samples from each training batch during the fine-tuning process. Results demonstrate significant acceleration by 45.6% in average across 6 models: GPT-2 S, GPT-2 M, DeBERTa-V2-XL, LLaMA-7B, LLaMA-2-7B, LLaMA-2-13B with average accuracy improvement by 5.91% in comparison to the fine-tuning results obtained with the use of LoRA method. ALOE (Offline) is able to accelerate GPT-2 M E2E-NLG fine-tuning by up to 92% with 1.2% BLEU improvement.