
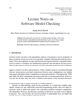
Non-autoregressive neural machine translation (NAT) has made remarkable progress since it is proposed. The performance of NAT in terms of BLEU has approached or even matched that of autoregressive neural machine translation (AT). However, other evaluation metrics show that NAT still lags behind. Unfortunately, these metrics only provide a numerical difference, and it is unclear how the translations produced by NAT differ from those produced by AT. In addition, the multimodality problem is always a significant issue in NAT. To assess whether NAT models are fully capable of solving the multimodality problem and achieving the performance of AT, we specifically design an error taxonomy to annotate errors in translations. The taxonomy is grounded on a systematic and hierarchical error analysis. We carry out an extensive annotation with professional annotators and analyze four NAT models and two AT models. Our analysis and experiments show that (1) the number of errors in NAT translations marked by annotators is 1.54 times that of AT translations, (2) the multimodality problem of NAT affects translations from lexical to syntactic levels, and even up to discourse, and (3) the four NAT models cannot fully eradicate the multimodality problem despite mitigation efforts.