
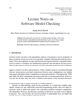
The wide spread of rumors with images and texts on social media has attracted broad attention in the academy and industry. Existing models focus on utilizing powerful feature extractors to obtain multi-modal features and introducing various external knowledge. However, the intrinsic semantic similarity of different modalities is either simply ignored in most models or far from adequate in others. The insufficiency of semantic similarity information suppresses the potential of rumor detection models severely. To address this issue, we propose a novel model termed the Semantic Similarity driven Multi-modal model (SemSim) for rumor detection, which deeply captures the semantic similarity through more comprehensive fusion between different modalities and designs a new classification method consequently. Specifically, the proposed SemSim first integrates the raw image and raw text into a virtual image, which fuses information at a new view, i.e., via the diffusion process inside stable diffusion models. Then SemSim captures the semantic similarity score between virtual image and raw image as the intrinsic information to drive SemSim. Besides, co-attention mechanism is employed to further perceive consistency and enhance interaction between the raw text-image pair. The fused representations via co-attention are utilized to evaluate the multi-modal feature score. In the end, SemSim balances the above two scores for final classification. Experiments on two typical real-world datasets show that SemSim can effectively detect rumors and outperform state-of-the-art methods.