
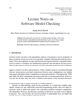
Automated persuasion systems (APS) are conversational agents that exchange arguments and counterarguments with users during dialogues to persuade them to believe in something. Such systems use strategies (or policies) to carefully select a sequence of arguments that are tailored to the user’s needs and will likely have a positive outcome, that is, changing the user’s belief in a certain argument. Biparty Decision Theory (BDT) is a framework that uses game theory to formalize a dialogue between an APS and a user, that is, an exchange of (counter) arguments during each turn of the APS or the user. During the APS turn, the BDT policy selects the best argument to maximize only the utility for the APS and neglects the utility of that argument for the user. This is a reasonable choice in games, but in a persuasive dialogue, it can result in arguments that have a high utility for the APS but a modest utility for the user. There the user may be less likely to be persuaded. This is crucial in settings where there are no arguments with good utilities for both the APS and the user and a compromise has to be found. To this extent, we define a new family of policies for BDT, called aggregated policies, that consider, during the decisions of the APS, an aggregation of the APS and user’s utilities. Such an aggregation considers both the APS and the user’s needs leading toward a sequence of arguments representing the best trade-off of utilities. We evaluate the approach using both a new synthetic dataset and a published dataset of utilities for dialogical argumentation. The results show the aggregated policies find better compromise arguments w.r.t. the classical policy of BDT.