
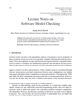
Graph Neural Networks (GNNs) learn the correlation between local graph neighborhoods and node properties, with the requirement of adequate complete and clean neighborhoods. However, the intrinsic structural characteristics of the long-tail node degree distribution in most real-world graphs violate the assumption. Numerous low-degree nodes lack accurate representation due to limited connections, while bits of high-degree nodes include redundancy and errors in observed neighborhoods, harming the performance of GNNs. In this paper, we propose Laner-GNN, which performs latent neighborhood restoration adapting to aforesaid structural characteristics and improves representation capacity. Specifically, to reduce computational complexity, latent disentangled neighborhood factors are extracted and then manipulated for restoration instead of explicitly altering the entire adjacency matrix. A Degree-Adaptive Restorer captures the dependency of restoration on node degrees, while a Label-Informed Restorer and the Structured Field Augmented Priori enhance restoration with information from the prediction target and the graph structure. Extensive experiments on real-world datasets in different domains verify that our model excels existing SOTAs in different degree bins, thus uplifting the model performance in the whole dataset.