
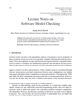
Fine-Grained Multimodal Named Entity Recognition and Grounding (FMNERG) aims to extract entity name, fine-grained entity type, and its corresponding object from paired text and image. This task demands fundamental reasoning capability for complex language and multimodal comprehension. Despite encouraging results, existing methods face two critical issues: (1) Insufficient knowledge of the entity poses challenges to fine-grained entity recognition; (2) Limited correlations between entities and objects hinder the visual grounding of entities. To tackle these issues, we propose a Multi-View Prompt (MVP) method for the FMNERG task in this paper, which collaborates with Large Language Models (LLMs) and Visual Grounding Models (VGMs) for reasoning. Concretely, MVP constructs a knowledgeable prompt in a chain-of-thought format, progressively refining possible entity types from coarse-grained to fine-grained levels. It leverages a heuristic method to select demonstration examples, which could provide guiding knowledge about entities from LLMs. To establish correlations between entities and potential objects, MVP introduces a grounded prompt that exploits information from guiding knowledge and image caption, enabling VGMs to detect related objects. Experimental results indicate that MVP achieves state-of-the-art performance on the Twitter dataset.