
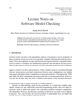
Fraud detection has attracted massive research interests due to its broad applications, especially on e-commerce websites, where fraudulent users interact with products unfairly to mislead potential customers for monetary incentives. Previous studies have made significant research progress on fraud detection, while only a few of them pay attention to adaptability and scalability of their methods. As a result, most of prior fraud detectors cannot perform consistently well across different scenarios due to issues such as sparsity, pattern inconsistency and camouflage. To address these issues, this paper proposes an adaptive algorithm called AdaptFD to effectively detect fraudulent users on e-commerce platforms. In particular, we first tackle sparsity issue with finer granularity by inferring relevant coefficients on entity level during runtime. Next, variable regularization terms are designed for pattern inconsistency tolerance. Lastly, we analyze the behavior patterns of users to reveal camouflage of fraudsters. Together, AdaptFD can adapt to different scenarios and handle with large-scale data efficiently. We also provide theoretical guarantees for our algorithm in linear time complexity and training convergence. Extensive experiments on four real-world datasets demonstrate that AdaptFD outperforms other state-of-the-art baselines. It’s worth noticing that our algorithm achieves up to 560x speedup on the real-world dataset, compared with the most related baseline.