
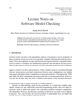
As Federated Learning (FL) gains prominence in secure machine learning applications, achieving trustworthy predictions without compromising predictive performance becomes paramount. While Differential Privacy (DP) is extensively used for its effective privacy protection, yet its application as a lossy protection method can lower the predictive performance of the machine learning model. Also, the data being gathered from distributed clients in an FL environment often leads to class imbalance making traditional accuracy measure less reflective of the true performance of prediction model. In this context, we introduce a fairness-aware FL framework (TrustFed) based on Gaussian differential privacy and Multi-Objective Optimization (MOO), which effectively protects privacy while providing fair and accurate predictions. To the best of our knowledge, this is the first attempt towards achieving Pareto-optimal trade-offs between balanced accuracy and fairness in a federated environment while safeguarding the privacy of individual clients. The framework’s flexible design adeptly accommodates both statistical parity and equal opportunity fairness notions, ensuring its applicability in various FL scenarios. We demonstrate our framework’s effectiveness through comprehensive experiments on five real-world datasets. TrustFed consistently achieves comparable performance fairness tradeoff to the state-of-the-art (SoTA) baseline models while preserving the anonymization rights of users in FL applications.