
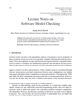
Federated learning enables multiple clients to collaboratively train a global model without revealing their local data. However, conventional federated learning often overlooks the fact that data stored on different clients may originate from diverse domains, and the resulting domain shift problem can significantly impair the performance of the global model. In this paper, we introduce Federated Semantic Prototype Learning (FedSeProto), a semantic prototype-based approach designed to address the domain shift issue in federated learning. The proposed method comprises two components: feature decoupling and feature alignment. Feature decoupling aims to learn semantic prototypes that can represent semantic information associated with specific categories, while feature alignment utilizes these semantic prototypes to facilitate learning of cross-client consistent features. Two key techniques are employed to achieve feature decoupling. On one hand, feature separation is achieved through the minimization of mutual information between semantic and domain features. On the other hand, the knowledge distillation is leveraged to ensure that both semantic and domain features carry the correct information. For feature alignment, intra-class semantic features are used to generate the local prototypes, which are further aggregated to the global prototypes. These global prototypes serve as guides during the local training process. Specifically, the local intra-class semantic features are driven to close to the corresponding global prototypes, thereby encouraging all clients to learn the globally consistent semantic features. Comprehensive experiments conducted on four challenging multi-domain datasets demonstrate the effectiveness of the proposed method compared with existing federated learning algorithms.