
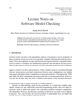
Multi-task learning (MTL) enables simultaneous learning of related tasks, enhancing the generalization performance of each task and facilitating faster training and inference on resource-constrained devices. Federated Learning (FL) can further enhance performance by enabling collaboration among devices, effectively leveraging distributed data to improve model performance, while ensuring that the raw data remains on the respective devices. However, conventional FL is inadequate for handling MTL models trained on different sets of tasks. This paper proposes FedMTL, a new FL aggregation technique that handles task heterogeneity across users. FedMTL generates personalized MTL models based on task similarities, which are determined by analyzing the parameters for the task-specific layers of the trained models. To prevent privacy leakage through these model parameters and to protect the privacy of the task types, FedMTL employs low-overhead algorithms that are adaptable to existing techniques for secure aggregation. Extensive experiments on three datasets demonstrate that FedMTL performs better than state-of-the-art approaches. Additionally, we implement the FedMTL aggregation algorithm using secure multi-party computation, showing that it can achieve the same accuracy with the plain-text version while preserving privacy.