
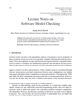
Heterogeneous dynamic graph neural networks (HDGNNs) are effective methods for processing heterogeneous temporal graphs (HTGs), which serve as ubiquitous data structures in real-world scenarios. The previous HDGNN paradigm obtains representations of future target nodes by mining the spatial heterogeneity and temporal dependence of node attributes, ignoring the learning of relation temporal dependence. However, through experience, we find that the learning of relation temporal dependence, which describes the evolving trends in the importance of neighbors under a certain relation, is beneficial for representation learning of HTGs. To bridge this gap, we propose a novel end-to-end heterogeneous temporal graph learning paradigm called Relation Time-aware Heterogeneous Dynamic Graph Neural Networks (ReTag). Compared to previous HDGNNs, ReTag extracts the temporal dependence of relations from historical relation information and the evolving node attributes to drive subsequent spatio-temporal representation learning. As far as we know, ReTag is the first attempt to perform learning of the temporal dependence of relation, which can generate a more effective representation for different downstream tasks of HTGs. The experimental results of different downstream tasks of HTGs based on multiple benchmark datasets show that ReTag can obtain obvious performance advantages compared with the sota baseline method.