
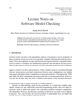
Graph Neural Networks (GNNs) have demonstrated remarkable capabilities across various domains. Despite the successes of GNN deployment, their utilization often reflects societal biases, which critically hinder their adoption in high-stake decision-making scenarios such as online clinical diagnosis, financial crediting, etc. Numerous efforts have been made to develop fair GNNs but they typically concentrate on either individual or group fairness, overlooking the intricate interplay between the two, resulting in the enhancement of one, usually at the cost of the other. In addition, existing individual fairness approaches using a ranking perspective fail to identify discrimination in the ranking. This paper introduces two innovative notions dealing with individual graph fairness and group-aware individual graph fairness, aiming to more accurately measure individual and group biases. Our Group Equality Individual Fairness (GEIF) framework is designed to achieve individual fairness while equalizing the level of individual fairness among subgroups. Preliminary experiments on several real-world graph datasets demonstrate that GEIF outperforms state-of-the-art methods by a significant margin in terms of individual fairness, group fairness, and utility performance.