
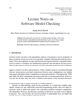
Recent approaches in hierarchical text classification (HTC) rely on the capabilities of a pre-trained transformer model and exploit the label semantics and a graph encoder for the label hierarchy. In this paper, we introduce an effective hierarchical text classifier RADAr (A Transformer-based Autoregressive Decoder Architecture) that is based only on an off-the-shelf RoBERTa transformer to process the input and a custom autoregressive decoder with two decoder layers for generating the classification output. Thus, unlike existing approaches for HTC, the encoder of RADAr has no explicit encoding of the label hierarchy and the decoder solely relies on the samples’ label sequences observed during training. We demonstrate on three benchmark datasets that RADAr achieves results competitive to the state of the art with less training and inference time. Our model consistently performs better when organizing the label sequences from children to parents versus the inverse, as done in existing HTC approaches. Our experiments show that neither the label semantics nor an explicit graph encoder for the hierarchy is needed. This has strong practical implications for HTC as the architecture has fewer requirements and provides a speed-up by a factor of 2 at inference time. Moreover, training a separate decoder from scratch in conjunction with fine-tuning the encoder allows future researchers and practitioners to exchange the encoder part as new models arise. The source code is available at unmapped: uri https://github.com/yousef-younes/RADAr.