
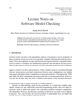
Online disease diagnosis, gathering the patients’ symptoms and making diagnoses through online dialogue, grows rapidly worldwide. Manual-based approach, e.g., Haodaifu, employs real-world doctors, providing high-quality but high-cost medical services. In contrast, machine-based approach, e.g., 01bot, that utilizes machine learning models can make automatic diagnosis but lacks reliable accuracy. While some work has enabled human-AI collaboration in disease diagnosis, their collaboration pattern is simple and needs to be further improved. Therefore, we aim to introduce a doctor-enhanced and low-cost human-AI collaboration pattern. There are two key challenges. 1) How to utilize expert knowledge in doctor feedback to enhance AI’s capability? 2) How to design a collaboration workflow to achieve a low-cost doctor workload while ensuring accuracy? To address the above challenges, we propose the Human-AI collaboration framework for disease diagnosis via doctor-enhanced transformer, called HAIformer. Specifically, to enhance AI’s capability, we propose a machine module that leverages doctors’ medical knowledge through doctor-enhanced attention, using a graph attention-based matrix; to reduce doctor workload, we propose an activation module that uses two units in a cascading manner for human-AI allocation. Experiments on four real-world datasets show that HAIformer can achieve up to 91.2% accuracy with only 18.9% human effort and one-third of dialogue turns. Further real-world clinic study highlights its advantages in practical applications.