
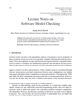
Traditional single-object tracking tasks are undergoing a new wave of transformation, especially with the emergence of the lack of semantics, which has led to the rise of the vision-language tracking task. However, previous approaches that combine the visual tracker with natural language descriptions tend to rely on a global representation of the text description, considering less about the fine-grained connections between the text description and the visual appearance. This paper proposes to utilize a bi-directional cross-attention module to capture the connections between language and visual features, which are further projected as dense semantic representations for alignment. In order to keep the semantic consistency between the search region and the coupled natural language and align the fused feature, this paper proposes a novel dense semantic contrastive learning loss to bridge the semantic gap between text and visual modalities and align them in a dense form. The proposed framework achieves promising results in tracking datasets that contain natural language descriptions, such as TNL2K, and OTB99-LANG. Our approach provides a novel solution for representing and aligning cross-modal information for the single object tracking task and may inspire further research in this field.