
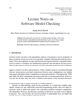
Weakly supervised semantic segmentation has attracted a lot of attention recently. Previous methods can be divided into two types, which are single-stage training and multi-stage training. In this paper, we focus on multi-stage training for image-level weakly supervised semantic segmentation. Many recent methods have tried to use transformer architecture as the backbone for CAM generation since it can capture global relationships to refine CAM accurately. However, we observe that such a backbone still fails to generate complete and smooth CAM. We argue that this is because the attention mechanism in the transformer can only pay attention to the most discriminative relationships. It is difficult to capture semantic-level long-range pair-wise relationships under image-level supervision. Thus, we propose an adversarial erasing transformer network called AETN, where an erasing attention mechanism is designed to establish more extensive pair-wise relationships. To cope with erasing, more target features will be forced to activate. Thus, better feature representation can be obtained for more accurate CAM generation. Besides, to further help our network learn better feature representation, we propose a self-consistent learning mechanism based on different augmentations. In this way, our AETN outperforms recent methods. Our AETN achieves 73.0 mIoU on the PASCAL VOC 2012 val set and 73.9 mIoU on the PASCAL VOC 2012 test set. Code is available a unmapped: uri https://github.com/siyueyu/AETN.