
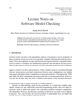
Market forecasting is crucial for the success of the commodity sales, and with the development of machine learning, a lot of companies are increasingly relying on data-driven models for market analysis and forecasting. In order to explore the application of machine learning for the commodity sales prediction, this paper constructs a product market prediction framework based on extreme gradient enhancement. Taking data-driven artificial intelligence machine learning as a method, it improves the accuracy and speed of predicting product sales in the market industry using mathematical modeling and manual analysis generally. Traditional manual analysis requires a long time and high cost to collect data, build models, and solve models among other processes. This article uses a Bayesian optimized limit gradient boosting intelligent prediction model to train and predict the ordering data of Zhenlong (Zhenpin) products in Guilin City in 2022 and predicts the sales of time series data and feature data respectively. The results showed that making full use of machine learning models for prediction has higher accuracy and faster speed comparing to traditional prediction methods, greatly optimizing the prediction process. This method provides a new research approach for predicting sales in the future commodity industry.