
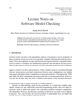
In response to the problem of excessive human intervention and poor accuracy of traditional methods for assessing the susceptibility of large-scale debris flow by selecting disaster-causing factors combined with statistical models, this paper proposes a DMANet (Dense-Multiscale-Attention Net) model for assessing the susceptibility of gully-type debris flows based on an improved Dense Net. Firstly, to improve assessment efficiency without reducing accuracy, the network structure of Dense Net is optimized. Secondly, the model uses an improved inception module to extract features of different scales to obtain local and overall features of the gully and integrates shallow features to reduce feature loss. Finally, to reduce the noise impact in gully images, the CBAM attention mechanism is introduced into the network, allowing the network to focus more on target samples. Based on the similarity between the gully to be assessed and the gully where debris flow has occurred, the susceptibility is predicted, and the results of susceptibility prediction are divided into five levels: extremely low susceptibility zone, low susceptibility zone, medium susceptibility zone, high susceptibility zone, and extremely high susceptibility zone using the natural breakpoint method. Experimental results show that the extremely high susceptibility zone of debris flow is mainly concentrated in the areas with abundant water systems on both sides of the Nu Jiang River, accounting for 56.53% of the entire study area, and the proportion of debris flow is 85.36%. The ACC value and AUC value of the DMANet model reach 77.43% and 0.7903 respectively, indicating that the model is a high-performance method for assessing the susceptibility of debris flow. This method can objectively and efficiently assess the susceptibility of debris flow, providing some ideas for the prevention and control of debris flow in the future.