
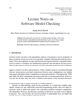
With the development of smart healthcare, ECG monitoring has become an integral part of remote health care and plays a crucial role in diagnosing arrhythmias. However, the current mainstream ECG automatic diagnosis models lack research on incremental learning with accumulated personal data. Therefore, this paper proposes a personalized incremental learning method for diagnosing arrhythmias to facilitate the development of individualized models for personal users. Initially, the individual’s ECG signals are encoded through ECG feature extractor composed of ResBlock, and Bi-LSTM. Subsequently, ECG diagnosis is performed using a personalized classifier tailored to the individual. As the personal data accumulates to a sufficient quantity, the personalized classifier is fine-tuned by incorporating the individual sample dataset with an arrhythmias-priority examplars based on herding, thus enabling the model to adapt to the individual domain. The experimental results demonstrate that the proposed model achieves an accuracy of 87.08% on the CPSC2018 dataset. Moreover, upon personalized incremental fine-tuning on the CPSC2020 dataset, the model’s performance improves by over 13% compared to the initial model. Hence, the proposed personalized incremental learning method is effective.