
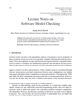
Large Language Models (LLMs) can appear to generate expert advice on legal matters. However, at closer analysis, some of the advice provided has proven unsound or erroneous. We tested LLMs’ performance in the procedural and technical area of insolvency law in which our team has relevant expertise. This paper demonstrates that statistically more accurate results to evaluation questions come from a design which adds a curated knowledge base to produce quality responses when querying LLMs. We evaluated our bot head-to-head on an unseen test set of twelve questions about insolvency law against the unmodified versions of gpt-3.5-turbo and gpt-4 with a mark scheme similar to those used in examinations in law schools. On the “unseen test set”, the Insolvency Bot based on gpt-3.5-turbo outper-formed gpt-3.5-turbo (p = 1.8%), and our gpt-4 based bot outperformed unmodified gpt-4 (p = 0.05%). These promising results can be expanded to cross-jurisdictional queries and be further improved by matching on-point legal information to user queries. Overall, they demonstrate the importance of incorporating trusted knowledge sources into traditional LLMs in answering domain-specific queries.