
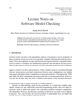
Skeleton-based action recognition algorithms have made extensive use of graph topologies to model the connections between human skeletal joints. Constructing graph topology models with greater representational power is key to obtaining powerful feature extractors. However, existing methods are not as effective in achieving stronger modelling of associations between physically connected joints in the human skeleton. To tackle these concerns, we put forward an innovative approach involving a distinct graph topology - a graph convolutional neural network (SGC-GCN) that amalgamates a dynamic, refined graph topology with a static, shared graph topology. The dynamic partial feature extractor (CTR-GC) and the static partial feature extractor (SGC-GC) are used in conjunction with each other to obtain a stronger feature aggregation capability in the form of static reinforcement of dynamic weaker features and to achieve the objective of strengthening the correlation modelling between interconnected joints within the human skeletal structure. The combined use of the two also introduces only a small number of additional parameters, ensuring that the refined features are not affected by the noise of statically shared features. Combining SGC-GC with the temporal modelling module has resulted in the development of SGC-GCN, a graphical convolutional network with even greater feature aggregation capability. Our network demonstrates a remarkable performance surpassing existing advanced methods on the dataset(NTU RGB+D), yielding substantial advancements in action recognition accuracy.