
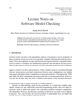
Customer care is an essential pillar of the e-commerce shopping experience with companies spending millions of dollars each year, employing automation and human agents, across geographies (like US, Canada, Mexico, Chile), channels (like Chat, Interactive Voice Response (IVR)), and languages (like English, Spanish). SOTA pre-trained models like multilingual-BERT, fine-tuned on annotated data have shown good performance in downstream tasks relevant to Customer Care. However, model performance is largely subject to the availability of sufficient annotated domain-specific data. Cross-domain availability of data remains a bottleneck, thus building an intent classifier that generalizes across domains (defined by channel, geography, and language) with only a few annotations, is of great practical value.
In this paper, we propose an embedder-cum-classifier model architecture which extends state-of-the-art domain-specific models to other domains with only a few labeled samples. We adopt a supervised fine-tuning approach with isotropic regularizers to train a domain-specific sentence embedder and a multilingual knowledge distillation strategy to generalize this embedder across multiple domains. The trained embedder, further augmented with a simple linear classifier can be deployed for new domains. Experiments on Canada and Mexico e-commerce Customer Care dataset with few-shot intent detection show an increase in accuracy by 20-23% against the existing state-of-the-art pre-trained models.