
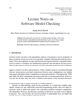
Due to the expensive segmentation annotation cost, cross-modality medical image segmentation aims to leverage annotations from a source modality (e.g. MRI) to learn a model for target modality (e.g. CT). In this paper, we present a novel method to tackle cross-modality medical image segmentation as semi-supervised multi-modal learning with image translation, which learns better feature representations and is more robust to source annotation scarcity. For semi-supervised multi-modal learning, we develop a deep co-training framework. We address the challenges of co-training on divergent labeled and unlabeled data distributions with a theoretical analysis on multi-view adaptation and propose decomposed multi-view adaptation, which shows better performance than a naive adaptation method on concatenated multi-view features. We further formulate inter-view regularization to alleviate overfitting in deep networks, which regularizes deep co-training networks to be compatible with the underlying data distribution. We perform extensive experiments to evaluate our framework. Our framework significantly outperforms state-of-the-art domain adaptation methods on three segmentation datasets, including two public datasets on cross-modality cardiac substructure segmentation and abdominal multi-organ segmentation and one large scale private dataset on cross-modality brain tissue segmentation. Our code is publicly available at https://github.com/zlheui/DCT.