
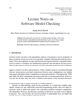
Knowledge-grounded dialogue (KGD) has become increasingly essential for online services, enabling individuals to obtain desired information. While KGD contains knowledge information, most knowledge points are fragmented and repeated in dialogues, making it difficult for users to quickly grasp complete and key information from a collection of sessions. In this paper, we propose a novel task of dialogue-grounded knowledge points generation (DialKPG) to condense a collection of sessions on a topic into succinct and complete knowledge points. To enable empirical study, we create TopicDial and OpenDial corpus based on two existing knowledge-grounded dialogue corpus FaithDial and OpenDialKG by a Three-Stage Annotation Framework, and establish a novel approach for DialKPG task, namely MSAM (Multi-Level Salience-Aware Mixture). MSAM explicitly incorporates salient information at the token-level, utterance-level, and session-level to better guide knowledge points generation. Extensive experiments have verified the effectiveness of our method over competitive baselines. Furthermore, our analysis shows that the proposed model is particularly effective at handling long inputs and multiple sessions due to its strong capability of duplicated elimination and knowledge integration.