
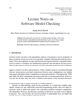
Knowledge graph completion (KGC, also referred to as link prediction) aims at predicting missing entities and relations in knowledge graphs (KGs). Knowledge graph embedding (KGE) techniques have been proven to be effective for link prediction. Currently, a series of convolutional neural networks (CNNs) based models (e.g., ConvE and its extended models) have attained excellent results for link prediction. However, several aspects that are important for link prediction using CNNs have not been considered and enhanced simultaneously, which significantly limit the performance of these models. In this paper we explore an effective KGE model based on CNNs. We investigate and discover four extremely important aspects that have a strong influence on ConvE: entity and relation embeddings, entity-to-relation interaction approaches, CNN structure, and loss function. Based on the optimization of the above four aspects, we propose a novel KGE method called ConvEICF. Through extensive experiments, we find that ConvEICF outperforms the previous state-of-the-art link prediction baselines on FB15k-237 and WN18RR datasets. In particular, ConvEICF achieves a Hits@10 score that is 11.2% and 6.5% better than ConvE on FB15k-237 and WN18RR datasets respectively. Additionally, through in-depth experiments we observe an interesting phenomenon and important finding that the very common 1-N scoring technique in KGE can be considerably improved by just adding a dropout operation. Our code is available at https://github.com/NEU-IDKE/ConvEICF.