
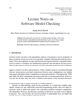
Class-incremental learning (CIL) has attracted much attention in deep learning due to the challenge problem of catastrophic forgetting. Various methods have been proposed for CIL, including exemplar-based class-incremental learning (EBCIL), non-exemplar class-incremental learning (NECIL) and data-free class-incremental learning (DFCIL). Without storing any information (such as examples and prototypes) about the old classes, DFCIL is obviously the most challenging one. To address the problem of lacking information in DFCIL and with the assumption that the learned representations are not linearly separable, we propose a method called IRP. We use the L2-similarity classifier instead of the FC classifier, where each weight vector represents a prototype that implicitly records information about the classes. We use representation-prototype distance minimization (RPDM) to solve the problem of loose representation caused by overfitting. To alleviate the excessive deviation of old prototypes under long-term CIL, we add prototype changing limitation (PCL) and prototype momentum updating (PMU) in incremental stages. In addition, we design a method for resampling around old prototypes (RAOP) to maintain the decision boundary of the old classes. Numerous experiments on three benchmarks have shown that IRP is significantly superior to other DFCIL methods and performs comparably to NECIL and partial EBCIL methods.