
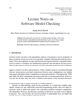
Unsupervised visual representation learning has gained much attention from the computer vision community because of the recent contrastive learning achievements. Current work mainly adopts instance discrimination as the pretext task, which treats every single instance as a different class (negative) and uses a collection of data augmentation techniques to generate more examples (positive) for each class. The idea is straightforward and efficient but will generally cause similar instances to be classified into different classes. Such problem has been defined as “class collision” in some previous works and is shown to hurt the representation ability. Motivated by this observation, we present a solution to address this issue by filtering similar negative examples from each mini-batch. Concretely, we model the problem as a Determinantal Point Process (DPP) so that similar instances can be filtered stochastically, and diverse samples are expected to be sampled for contrastive training. Besides, we further introduce a priority term for each instance, which indicates the hardness of its positives, so that instances with more hard positives are more likely to be sampled for contributing to the optimization. Our sampling can be efficiently implemented in a feed-forward manner and further accelerated by our encouraged complement DPP. Extensive experimental results demonstrate our priority over the standard setup of contrastive learning.