
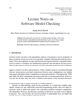
Classifying and segmenting patterns from a limited number of examples is a significant challenge in remote sensing and earth observation due to the difficulty in acquiring accurately labeled data in large quantities. Previous studies have shown that meta-learning, which involves episodic training on query and support sets, is a promising approach. However, there has been little attention paid to direct fine-tuning techniques. This paper repurposes contrastive learning as a pre-training method for few-shot learning for classification and semantic segmentation tasks. Specifically, we introduce a generator-based contrastive learning framework (GenCo) that pre-trains backbones and simultaneously explores variants of feature samples. In fine-tuning, the auxiliary generator can be used to enrich limited labeled data samples in feature space. We demonstrate the effectiveness of our method in improving few-shot learning performance on two key remote sensing datasets: Agriculture-Vision and EuroSAT. Empirically, our approach outperforms purely supervised training on the nearly 95,000 images in Agriculture-Vision for both classification and semantic segmentation tasks. Similarly, the proposed few-shot method achieves better results on the land-cover classification task on EuroSAT compared to the results obtained from fully supervised model training on the dataset.