
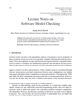
This paper proposes a simple-yet-efficient model, called capsule network with label dependency modeling (CapsLDM), for the task of multi-label emotion classification (MLEC) in text, in which multiple emotion categories can be assigned to the input data instance (e.g., a sentence). Unlike the traditional single-label emotion classification, the modeling of label (i.e., emotion) dependency plays an important role in MLEC, since the co-existing emotions in an utterance are not independent of each other. The capsule network has been successfully applied to many multi-label classification scenarios, however, the modeling of label dependency has not been considered in existing work. In our proposed CapsLDM model, we add similarity regularization terms on both the dynamic routing weights and the instance vectors of emotion capsules by exploiting the co-occurrence information of emotion labels, which resembles the dependency between different emotion categories for a certain input instance. Extensive experiments are conducted on four MLEC benchmark datasets with state-of-the-art models employed as baselines for comparison. The empirical results demonstrate the superiority of our proposed CapsLDM model and confirm the effectiveness of label dependency modeling in CapsLDM for the MLEC task.